In order to achieve the goal of autonomous driving, ADAS applications are in full swing, and the demand for microcontrollers, sensor chips, and graphics processors built into various smart cars is increasing, which in turn drives semiconductor manufacturers to adopt car codes, high performance and high integration. product.
This article refers to the address: http://
Achieved self-driving vision car supply chain industry mobilization
In the Demolition Man film released in 1993, almost all cars were self-driving, but of course they were all concept cars. Today, more than ten leading automakers (including Audi, BMW, GM, Tesla, Foss and Volvo) are developing driverless cars. In addition, Google has also developed its own car technology, which has recently successfully traveled more than 1 million miles (equivalent to 75 years of average American adult driving) without major accidents.
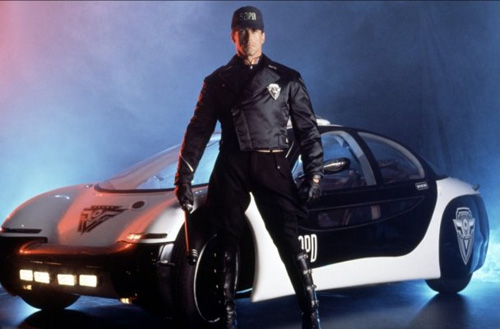
The prototype of the automatic concept car has appeared in the movie super-war. Look at these compelling results. Many people may ask a question: In the future, can we really see the driverless car driving on the road?
To achieve this vision, a complex automotive supply chain consisting of semiconductor operators, system integrators, software developers and automakers, all of which work closely together to develop key advanced driver assistance systems (ADAS) technologies. For the first commercial driverless car. Designed for electromechanical integrated motor control solutions, this embedded power IC is suitable for a wide range of motor control applications, and must be packaged in a small form factor (SFF) with minimal external components.
Import ADAS three design methods
There are three main ways to design the ADAS function in a car.
One approach is to allow cars to be used to navigate in specific environments by storing large amounts of map data. This method is like having a train run on an invisible track. One example of this approach is Google's driverless car, which uses a set of pre-recorded street HD maps to navigate, rarely using sensing technology. In this example, the car relies only on high-speed communication connections and sensors, and maintains a stable connection to the cloud architecture to provide the navigation coordinates it needs.
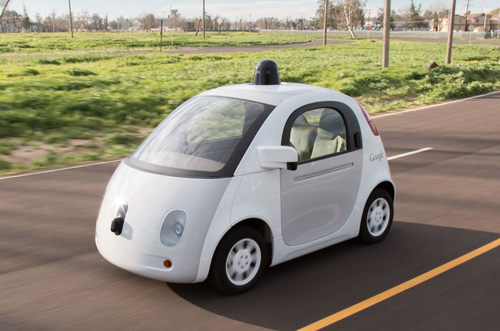
Google's driverless cars use a lot of map data for navigation, and the other technology uses computer vision processing, which is very dependent on prefabricated maps. This approach replicates the pattern of human driving because the car can make real-time decisions based on built-in multiple sensors and high-performance processors. This type of car typically includes multiple cameras that can be viewed from a distance and uses a special high-performance, low-power chip to provide supercomputer-level processing to execute ADAS software and hardware algorithms.
In the case of Mobileye, the company has been a pioneer in this technology, and its powerful and energy-efficient EyeQ SoC is designed for driverless cars. For example, the MIPS-based Mobileye EyeQ3 SoC is enough to support the required processing power for the highway auto-navigation feature recently offered by Tesla Electric. In this case, Mobileye uses a multi-core, multi-threaded MIPS I-class CPU to process data streams from multiple cameras in the car.
In the figure below, the four-core, four-threaded interAptiv CPU in the EyeQ4 SoC acts as the brain of the chip, directing the flow of information from the camera and other sensors to the right side of the chip, processing VLIW function blocks up to 2.5 TFLOPS.
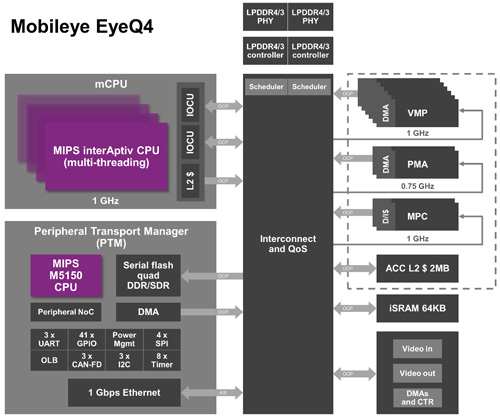
The EyeQ4 SoC has a quad-core, four-threaded interAptiv CPU. The third trend is to develop a driverless car with a general-purpose SoC processor. For cars that already have an embedded GPU to support the infotainment system, developers can use the computational resources of the graphics engine to perform various identification and tracking algorithms for lanes, pedestrians, cars, building facades, parking lots, and more. For example, Luxoft's computer computing and augmented reality solutions use Imagination's PowerVR image architecture and additional software to build ADAS functionality.
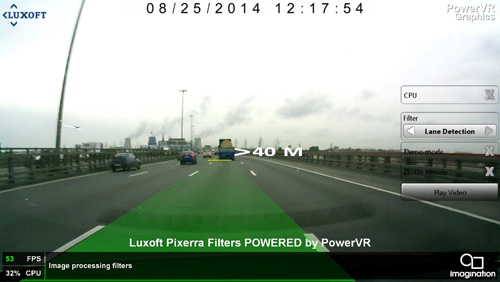
PowerVR image architecture quickly builds a variety of in-car autopilot features
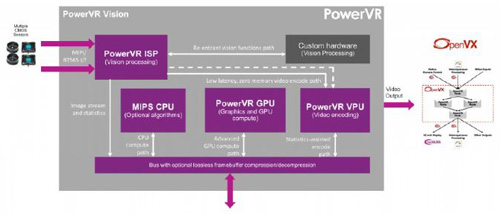
PowerVR Series7 GPU Schematic This software architecture has been optimized for embedded PowerVR hardware to help quickly and cost-effectively implement a variety of in-car autopilot features.
Self-driving will bring unlimited business opportunities
Regardless of the design approach, driverless cars can open the bright future for automotive manufacturers, sensor suppliers, and the semiconductor industry. These vehicles will benefit from advanced SoC modules with hardware memory management, multi-threading and virtualization capabilities, and high-performance microcontrollers. These capabilities allow OEMs to build more complex software applications, including model-based process control, artificial intelligence, and advanced visual computing.
Distribution Box
Distribution Box
Wenzhou Korlen Electric Appliances Co., Ltd. , https://www.zjaccontactor.com